Data Innovations in Healthcare: Sometimes Two Steps Forward Requires One Step Back
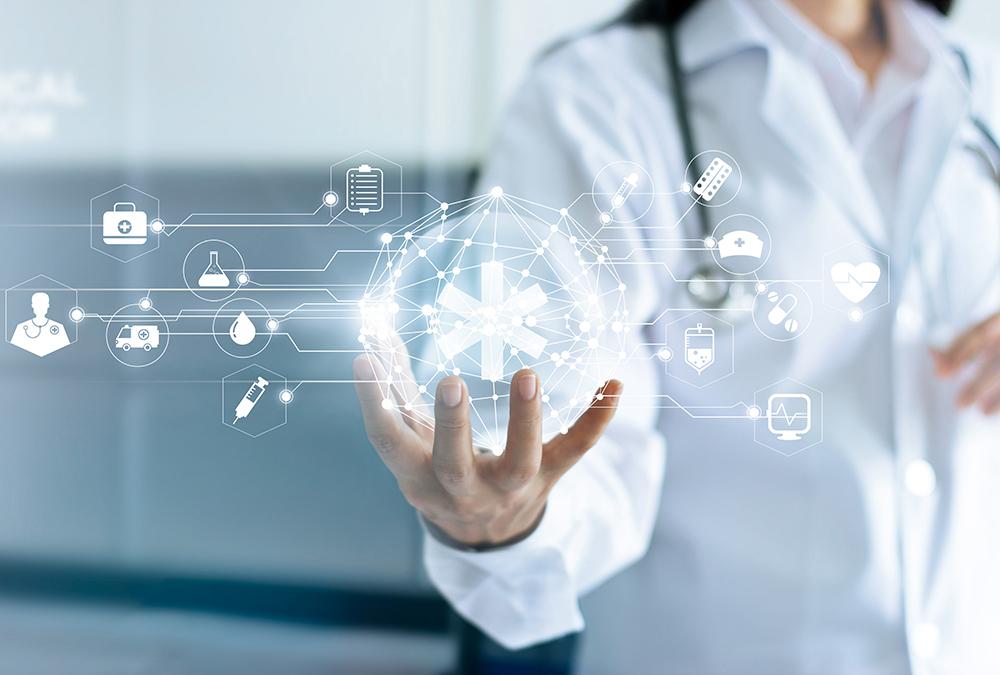
Technological innovations in the storage and computing capacity in the world of data has moved exponentially – especially in the healthcare space. One of the most dramatic use cases is in the field of genomics. As Andrea Norris, the CIO for NIH mentioned at a public sector Healthcare Summit on IT Modernization last week, technology has greatly increased the speed and lowered the costs associated with gene sequencing. Originally, when the human genome project completed the task, it took around 13 years with funding around 2.1 billion dollars (the project was from 1990–2003). From 2007–2009 costs went down from $10 million to $100k and processing took approximately 14 days. Now it costs in between $1500 to $1000 and can be done in 26 hours. This data creates huge data management challenges, but the benefits to accelerate cures for diseases are immense.
At the 2019 AFCEA Heath IT Summit on Modernization, data and innovation were central topics of focus. From data protection, moving to the cloud, analytics, machine learning and AI, the criticality of data as an asset to drive innovation in healthcare services and better patient outcomes was repeated across public sector speakers from CMS, DHA, HHS, HRSA, NIH, and the VA. Across the breakout sessions, a growing consensus among public sector leaders also emerged around data “plumbing” as a key road block to leveraging data as a strategic asset for innovation. Before advancing mature data capabilities, many agencies still struggle to get the basic infrastructure and processes in place.
What are some of the plumbing issues requiring mitigation? Not surprisingly, data management and integration are at the top of the list – especially the handling of vast amounts of diverse, expanding structured and unstructured data formats. Jude Soundararajan for example, who is the Health IT Director at SSA, noted that his organization handles over 15 million written paper records. Before the value from such information can be extracted, these assets have to be scanned, imaged, and viewed. Each steps poses a data management challenge. The quest to structure unstructured data can overwhelm, but his organization is using Optimal Character Reference tools (OCR) – leveraging AI tools such as natural language processing (NLP) methods – to synthesize the information. Using pattern recognition and computer vision tools could also facilitate prompt evaluation and analysis of patient records. Streamlining these workloads to scale and add value on-demand however requires a modern data infrastructure.
Getting back to the basics also involves process and the need to create data governance structures within an organization. Col. John Scott, Data Manager at DHA, knows firsthand the complications of siloed information stemming from the growth of insular departmental data hubs. These data are difficult to integrate if standards and quality have not been governed from an enterprise wide perspective. He has been successful using partnerships and collaboration to overcome these familiar obstacles – including interagency data sharing initiatives. Through the use of a data governance board, the DHA has been able to collaborate with the VA to share data – over 20 million patient records. Much of this data is unstructured and requires new infrastructure not only to store, but manage and process. Computing capacity is a current challenge for Col. Scott and his team of data scientists across DHA. Understanding from an enterprise level what data you have, how it will be used and who should have access are key to driving a single source of truth and the infrastructure an organization requires to scale and grow data services across a set of diverse stakeholders.
Interoperability complicates the process of optimizing patient services and innovations in predictive health as Dr. Les Folio, Senior Research Physician at NIH knows all too well. The varied sources stemming from different systems used to track and manage a care recipient’s medical journey over time, creates a storage capacity issue, and difficulties for any physician hoping to secure a 360 degree view of their patients. The complexity associated with analyzing the abundance of data, much of it images, creates storage capacity issues as well. The need for an enterprise data strategy to overcome these data management issues is critical. Despite these challenges, Dr. Folio has created a simulated AI workflow, using machine learning to detect tuberculosis in x-rays, which has produced over 37% resource optimization (time taken to diagnose) and improved quality in detection capabilities. This type of performance impact (creating resource capacity) should be leveraged to create a business case to further agency investments in next generation infrastructure to scale data management and computing requirements.
Large amounts of structured and unstructured data, siloed information systems and interoperability are all issues facing many agencies as they seek to mature their respective data programs. The quest to pilot and then roll out advanced data solutions, including AI workflows and other optimization techniques, which improve operations, customer experiences and ROI require organizations to go back to the basics.
DLT has technology partners that drive the basics and catapult modernity to any agencies data strategy and programs.